FEATURE
Robot dogs take a walk on the wired side
Robots are learning to walk and work. While robot dogs are not yet man's best friend, real autonomy and reasoning will make them useful companions in industry, search & rescue and even space exploration, writes Tom Cassauwers.
The first chords of the 1960s Motown song Do You Love Me, by the Contours sound on the speakers as the robots start to dance. Several models, including a bipedal humanoid version, and a four-legged dog-like contraption, are seen dancing with each other. They shuffle, do pirouettes and swing.
Released by the US robotics company Boston Dynamics, the viral video of robots with legs dancing created a stir at the end of 2020. Reactions ranged from people suggesting it was made using CGI, to fear that the robots were going to take over the world. Yet for all the impressive engineering, the video also showed the limitations that legged robots face. Whereas for humans dancing is quite easy, for robots it's incredibly hard, and the three-minute video meant that every movement of the robots had to be manually scripted in detail.
'Today robots are still relatively stupid,' said Marco Hutter, professor at ETH Zurich and expert in robotics. 'A lot of the Boston Dynamics videos are hand-crafted movements for specific environments. They need human supervision. In terms of real autonomy and reasoning, we're still far away from humans, animals or what we expect from science-fiction.'
In terms of real autonomy and reasoning, we're still far away from science-fiction.
Marco Hutter, LeMo
Yet these sorts of robots could be very helpful to humanity. They could help us when disasters strike, they could improve industrial operations and logistics and they could even help us explore outer space. But for that to happen we need to make legged robots better at basic tasks like walking and teach them how to do so without supervision.
Virtual learning
The ERC-project LeMo is one of the investigations launched by European researchers to make robots move more autonomously. Their core premise is that legged locomotion isn't what it could be, and that machine learning techniques could improve it. LeMo is specifically focused on so-called reinforcement learning.
'Reinforcement learning uses a simulation to generate massive data for training a neural network control policy,' explained Hutter, who is also the project leader of LeMo. 'The better the robot walks in the simulation, the higher reward it gets. If the robot falls over, or slips, it gets punished.'
The robot they use in the project is a 50 kilogram, dog-like, four-legged robot. On top of it are several sensors and cameras that allow it to detect its environment. This part has become pretty standard for legged robots, yet the advancement LeMo produces lies in the software. Instead of using a model-based approach, where the researchers program rules into the system, like 'when there's a rock on the ground, lift up your feet higher', they 'train' an AI-system in a simulation.
Here the robot's system walks over and over through a virtual terrain simulation, and every time it performs well it receives a reward. Every time it fails it receives a punishment. By repeating this process millions of times, the robot learns how to walk through trial-and-error.
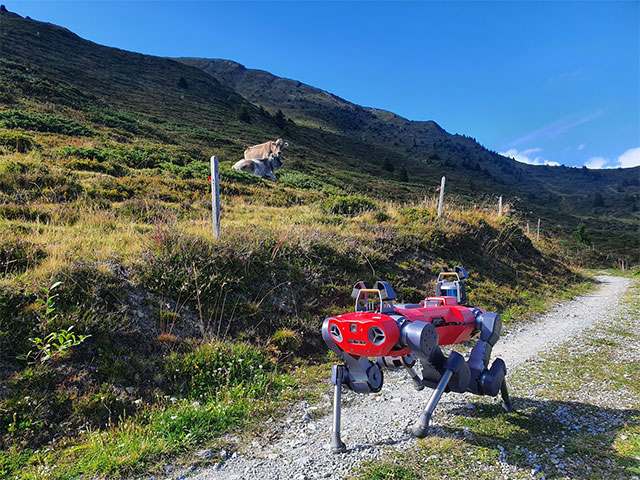
The LeMo project’s dog-like robot is one of the first to have learned to walk through reinforcement learning. ©ETH Zurich
'LeMo is one of the first times reinforcement learning has been used on legged robots,' said Hutter. 'Because of this, the robot can now walk across challenging terrain, like slippery ground and inclined steps. We practically never fall anymore.'
Using this technology, the ETH Zurich team recently won a $2 million Defense Advanced Research Projects Agency (DARPA) contest in which teams were challenged to deploy a fleet of robots to explore challenging underground areas by themselves.
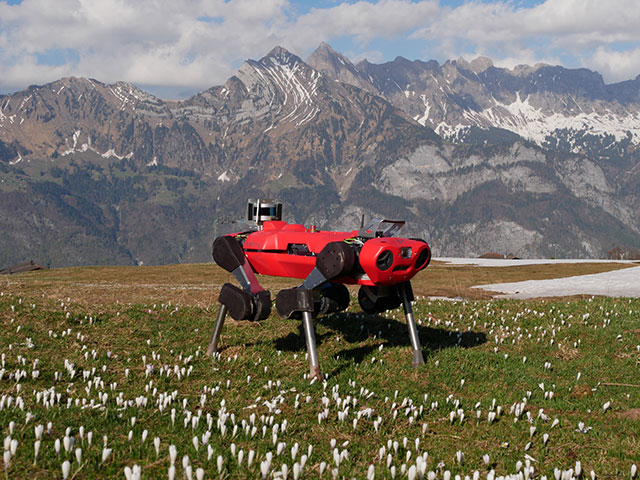
Robots with improved locomotive abilities can help in search and rescue operations and space exploration. ©ETH Zurich
'Legged robots are already used for industrial inspections and other observation tasks,' said Hutter. 'But there are also applications like search & rescue and even space exploration, where we need better locomotion. Using techniques like reinforcement learning we can accomplish this.'
Watch the video
Research in this article was funded via the EU’s European Research Council. If you liked this article, please consider sharing it on social media.